The Importance of Applied Ethics in Artificial Intelligence
Human data, the fundamental building block for artificial intelligent systems, is currently being accessed and shared in multiple ways across the world without unified policy, technology, or cultural guidelines. For industries like financial services — which is second only to the technology industry in AI adoption — that provide products and advice directly impacting the lives of their customers, it is imperative to bring abstract concepts of ethics, trust, and fairness into practical tools to foster fair, accountable, and transparent use of AI systems at scale.
“Upholding customer privacy and trust is paramount. The acceleration of digital and AI technologies makes it increasingly important and complex. It is critical to install practical and scalable approaches across the AI development lifecycle to align with corporate values, customer expectations, and regulatory requirements. The right balance between risk and innovation will accelerate AI adoption in a responsible way.”
– Dr. Ren Zhang, Chief Data Scientist and Head of AI Center of Excellence, BMO
Artificial Intelligence Systems in Financial Services
A sample of AI-driven applications broadly used in financial services where ethical design practices are beginning to be implemented include:
- Credit Decisions: AI systems solutions are helping banks and credit lenders make smarter underwriting decisions by utilizing a variety of factors that more accurately assess traditionally underserved borrowers, like millennials, in the credit decision making process.
- Managing Risk: Financial markets are turning more and more to machine learning, a subset of artificial intelligence systems, to create more exacting, nimble models. These predictions help financial experts utilize existing data to pinpoint trends, identify risks, conserve manpower and ensure better information for future planning.
- Personalized Banking: AI assistants, such as chatbots, use AI systems to generate personalized financial advice and natural language processing to provide instant, self-help customer service.
In combination with applications and practices reflecting AI ethics, countries like Canada have been among the first to prioritize human-centric, values-driven ethical methodologies for the application of AI systems, for example, with their creation alongside France of the International Panel on Artificial Intelligence. Building from a basis of fully disclosed, customer-centric data access and portability for customers, multiple financial institutions are recognizing that it is only by eliminating discriminatory bias or by increasing transparency that genuine trust with customers can flourish in the algorithmic age.
IEEE Finance Playbook for AI Ethics
In today’s financial services industry, everyone leading the space also relies on the services and offerings that banks provide. How we know our money is safe determines how we take care of our families and lead our lives. That’s why consensus from within the industry and community is so critical when it comes to Artificial Intelligence Systems.
To further address the need to bring AI ethical practices to applications in the financial services industry, over fifty industry thought-leaders from banks, credit unions, pension funds, law firms, academia, and technology services organizations based out of Canada, the US, and the UK, have created the first version of The IEEE Finance Playbook that provides a roadmap for Trusted Data and Artificial Intelligence Systems (AIS) for Financial Services.
Bringing together diverse voices from the finance and data sciences communities, The Playbook aims to build industry consensus on pressing ethical and societal issues, help financial organizations evaluate their trusted data and AI systems readiness, and create the foundation for customer confidence in AI.
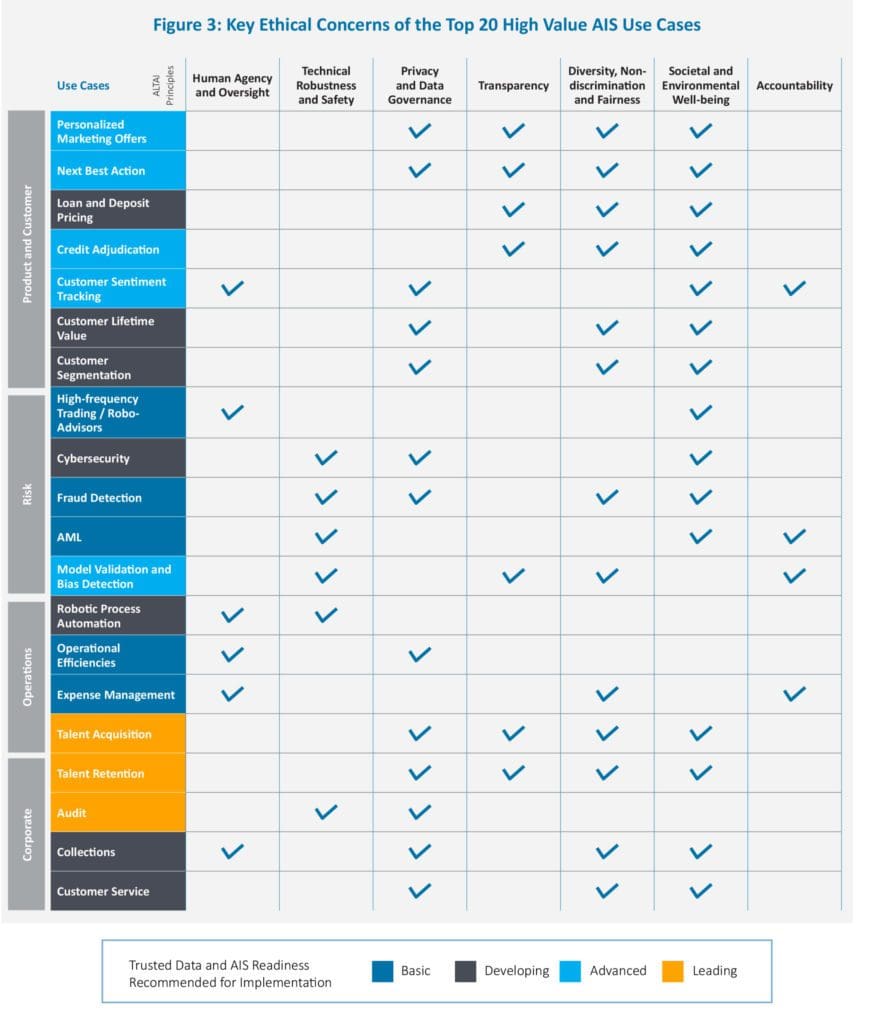
The Roadmap Toward Ethical AI in Finance
There are three sets of critical building blocks that allow organizations to develop, execute, and scale trusted data and AI systems as a key part of the Roadmap towards trusted AI Systems in finance:
- People: Operational efforts focused on technology and process alone are not enough to ensure trusted data and AI systems. Leading organizations also must focus on developing people-critical building blocks: a strong data and AI climate, leadership buy-in, a clear data and AI strategy, and education and awareness across the organization on the trusted data and AI initiatives.
- Process: In addition to technology-critical building blocks, leading organizations have in place trusted data and AI processes; specifically, a series of data and AI ethics governance tools including principles, data, AI impact assessments, as well as measurements and KPIs. Standards and certifications such as IEEE 7010 standards family and The Ethics Certification Program for Autonomous and Intelligent Systems (ECPAIS) are also starting to appear. These efforts will allow organizations to certify both their technical and governance initiatives for trusted data and AI systems.
- Technology: Institutions must have the appropriate technology-critical building blocks to scaling trusted data and AI projects. Through our studies, we have observed there to be a consistent scaling process that leads to the successful implementation of value-driving AI projects.

Based on an organization’s development across each of these three sets of critical building blocks, it will fall into one of four trusted data and AI systems readiness categories:
- Basic organizations may not yet have started or will have just embarked on the evolution of their business architecture using AI systems.
- Developing organizations will have started the trusted data and AI journey and will likely have some core technology scaling capabilities, along with a strong data and AI climate.
- Advanced organizations have taken several steps to evolve their business architecture using AI systems, and most notably have strong leadership buy-in, principles, in addition to a strong climate and developing technical scaling capabilities.
- Leading organizations are well on their way to transitioning to a new business architecture powered by AI systems, with trusted data and AI ethics initiatives in place across each of the three critical building blocks.
Examples of AI Ethics in Finance
The use of AI systems has helped organizations deliver incremental value through several avenues including engaging customers, increasing share of wallet, customer acquisition, reduced credit loss, and improved profitability. The IEEE Finance Playbook studies the top twenty value-delivering use case studies of trusted AI for financial services.
For example, when it comes to credit adjudication, AI can help us gain better insights into customer risk and make better, streamlined adjudication decisions. However, the design and implementation of AI systems should only come after the ethical issues are addressed.

It’s also critical to recognize that AI technology is in early stages in regards to how data is acquired and what data sets reflect local, regional, or international regulation. The “Trusted Data and AI Implications” are not only about “ethics” or Public Relations. These are foundational issues affecting how design and operations of any AI systems in its full value chain and systems development needs to be prioritized.
Along with additional use cases, this playbook also introduces the IEEE documents, standards, and programs that helped create and evolve this work as well as offers a deep dive into a Canadian perspective on how to address the post-pandemic world in regards to the key role financial services and AI systems can play.
How You Can Get Involved
While the thought leaders who put together the IEEE Finance Playbook understand the academic or business implications behind the technical aspects of AI systems, “transparency,” customers don’t. They are relying on experts to safeguard their data and assets to restore, gain, and maintain their trust. This will only happen by having a unified effort within the Finance and AI systems world to proactively and specifically tackle issues like bias, disclosure, and data access in ways that can be made clear to customers.
Authors:
- Cindy Pham, Senior Manager, AI Risk, Scotiabank, Editor of The IEEE Finance Playbook
- Stephanie Kelley, Ph.D. Candidate in Management Analytics and AI Ethics, Smith School of Business at Queen’s University, Editor of The IEEE Finance Playbook
- John C. Havens, Executive Director, IEEE Global Initiative on Ethics of Autonomous and Intelligent Systems, Editor of The IEEE Finance Playbook