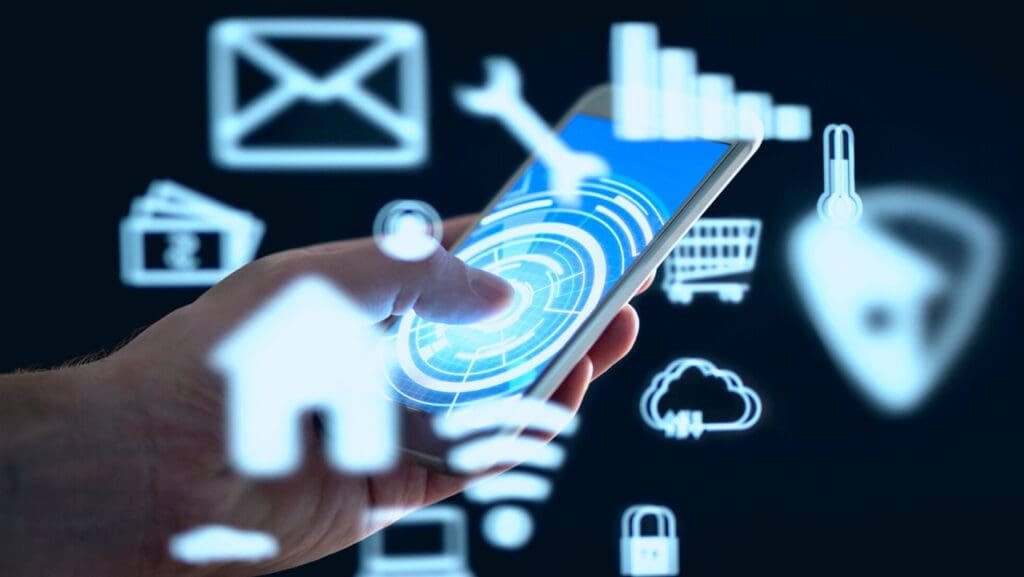

Brought to you by IEEE Standards Association (IEEE SA), Beyond Standards is a blog dedicated to promoting technology standards and celebrating the collaboration of individuals and organizations across the globe who drive technology development.
Contributions by IEEE SA’s global network of volunteers, members, staff, and partners, makes Beyond Standards a trusted source of information, education, and inspiration for industry, government, academia, and the public. We share updates and insights on a vast array of topics, focused on but not limited to: